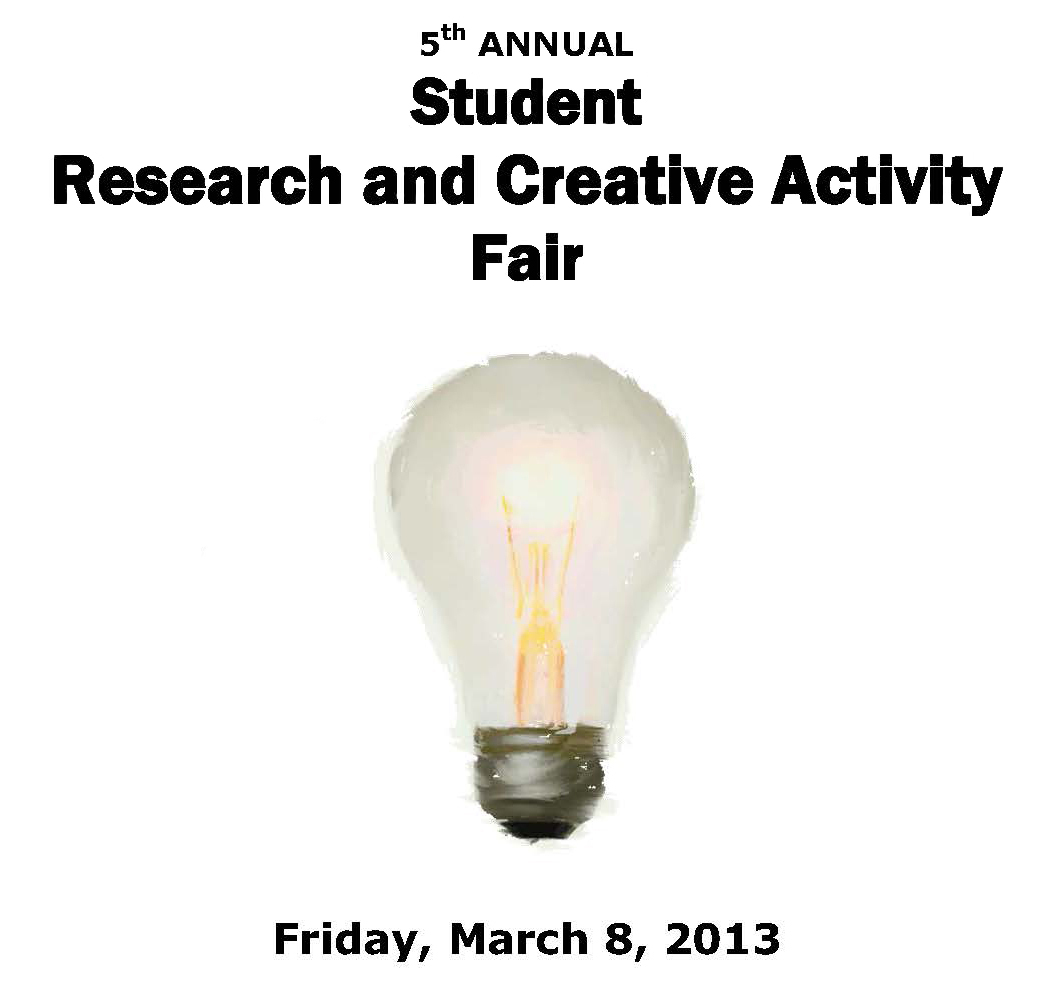
A Computational Model for Identifying Causative Genetic Relationships using Biological Networks and Gateway Nodes
Advisor Information
Hesham Ali
Location
Milo Bail Student Center Omaha Room
Presentation Type
Oral Presentation
Start Date
8-3-2013 11:30 AM
End Date
8-3-2013 11:45 AM
Abstract
Recently, High-throughput instruments and associated studies have produced volumes of publicly available metadata that provide a wealth of information that can be used to guide biological research. However, models for identifying actual signals and obtaining useful genetic relationships from such massive data have not been developed at the same rate. This is due in part to high levels of noise in the raw data and the lack of adequate algorithms for analysis. Thus, biological networks have emerged as a popular tool for modeling heterogeneous data because they are capable of representing biological relationships en masse. Moreover, well-established graph theoretic methodology can be applied to network models to increase efficiency and speed of analysis. We highlight how network representation of multiple states of biological data via correlation of gene expression allows us to formally define “Gateway” nodes. Gateway nodes can be loosely described as elements that connect clusters of genes between different states. We provide evidence that these nodes tend to represent genes that are critical for a shift from one cellular state to another. Further, we provide a proof-of-concept for the existence of these elements by mining gateway genes related to phenotypic shifts in yeast, mice, and humans. We verify these nodes as indicators of cellular activity using literature and knowledgebase derived from in vivo studies. The proposed computational model paves the way to establish novel ways to model and interpret gene expression data along with other biological data for the improvement of target identification in cellular systems.
A Computational Model for Identifying Causative Genetic Relationships using Biological Networks and Gateway Nodes
Milo Bail Student Center Omaha Room
Recently, High-throughput instruments and associated studies have produced volumes of publicly available metadata that provide a wealth of information that can be used to guide biological research. However, models for identifying actual signals and obtaining useful genetic relationships from such massive data have not been developed at the same rate. This is due in part to high levels of noise in the raw data and the lack of adequate algorithms for analysis. Thus, biological networks have emerged as a popular tool for modeling heterogeneous data because they are capable of representing biological relationships en masse. Moreover, well-established graph theoretic methodology can be applied to network models to increase efficiency and speed of analysis. We highlight how network representation of multiple states of biological data via correlation of gene expression allows us to formally define “Gateway” nodes. Gateway nodes can be loosely described as elements that connect clusters of genes between different states. We provide evidence that these nodes tend to represent genes that are critical for a shift from one cellular state to another. Further, we provide a proof-of-concept for the existence of these elements by mining gateway genes related to phenotypic shifts in yeast, mice, and humans. We verify these nodes as indicators of cellular activity using literature and knowledgebase derived from in vivo studies. The proposed computational model paves the way to establish novel ways to model and interpret gene expression data along with other biological data for the improvement of target identification in cellular systems.
Additional Information (Optional)
Winner of Best Graduate Oral Presentation