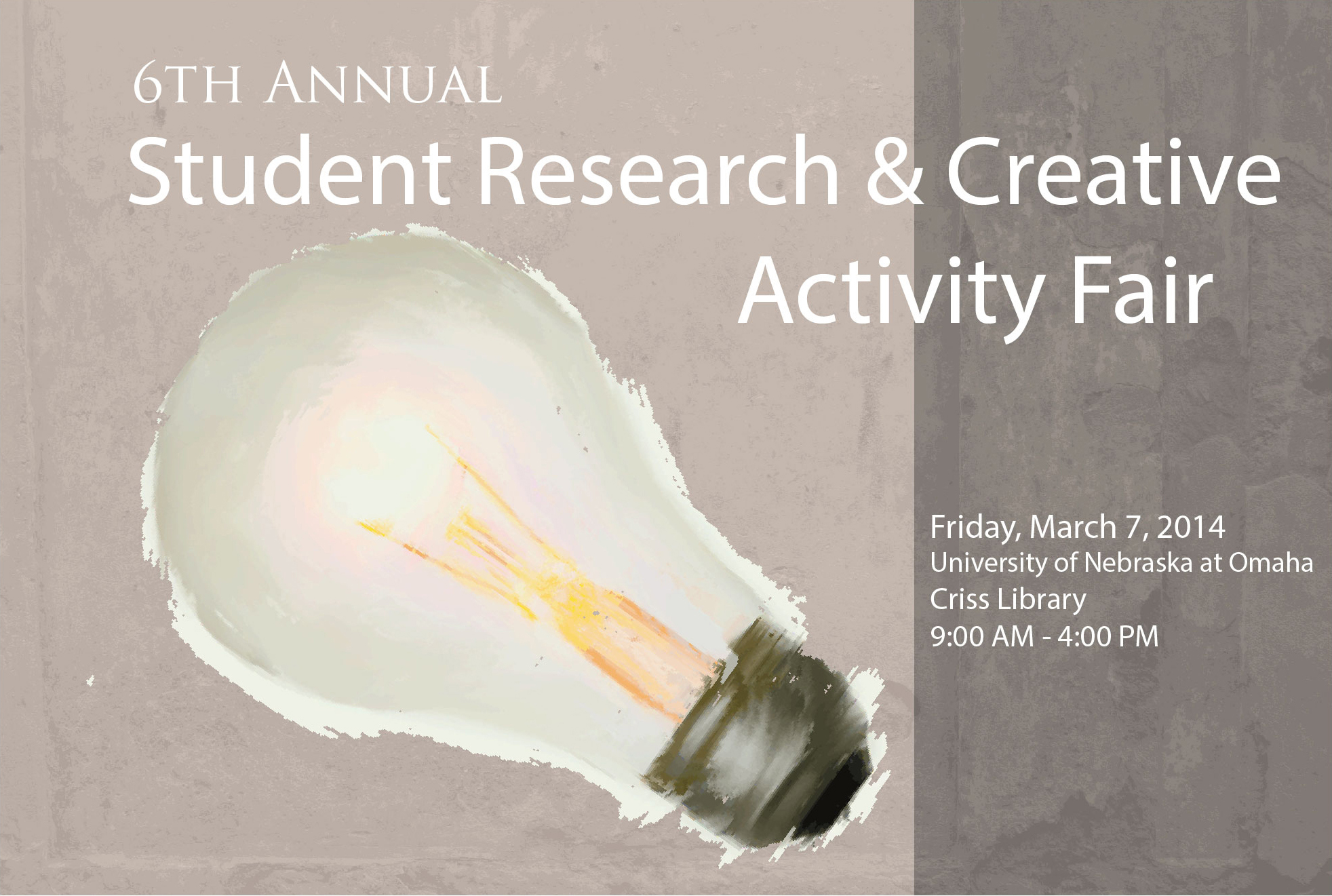
Integration of Domain Knowledge and Gene Expression Data in the Development of Enriched Correlation Networks
Advisor Information
Hesham Ali
Location
UNO Criss Library, Room 232
Presentation Type
Oral Presentation
Start Date
7-3-2014 1:30 PM
End Date
7-3-2014 1:45 PM
Abstract
The ability to model intragenic relationships using networks has allowed for the interpretation of considerable amounts of data, taking a key role in realization of systems biology. Practically, the use of gene correlation networks has assisted in the discovery of drugs as well as the illumination of previously unknown genetic relationships. Such networks provide a useful mechanism to model experimental results obtained from gene expression and capture a snapshot of the expression as well as the correlation of the experimental samples. Due to the fact that the noise to signal ratio in most biological databases are non-trivial, standard correlation networks may suffer from relatively high false-positive and false negative rates. Developing biologically-rich network enrichment algorithms can play a significant role in providing a healthy bias in the network and lead to the extraction of meaningful results. In addition, structure-based network filters can be used to reduce the network size and keep significant edges likely associated with strong biological signals. In this project, we propose the use of domain knowledge, not simply as an assessment tool, but as a basic component in building the correlation networks. We implemented a network integration algorithm that uses both gene expression data (experimental knowledge) and gene ontology data (domain knowledge) to build a biologically-rich correlation model. Our main hypothesis is that the integrated networks would reduce the harmful effects of outliers from imperfect data while maintaining the high concentration of network substructures that are likely to reveal novel, biologically-significant relationships. In addition, using the concept of “guilt by association”, we analyzed the clusters of the integrated networks and found that there was a significant increase of enrichment scores relative to the original networks. We also show higher concentration of known biological motifs calculated in the enriched networks. Based on the results obtained so far, the effects of outliers have been diminished in the new networks without the loss of the novel relationships.
Integration of Domain Knowledge and Gene Expression Data in the Development of Enriched Correlation Networks
UNO Criss Library, Room 232
The ability to model intragenic relationships using networks has allowed for the interpretation of considerable amounts of data, taking a key role in realization of systems biology. Practically, the use of gene correlation networks has assisted in the discovery of drugs as well as the illumination of previously unknown genetic relationships. Such networks provide a useful mechanism to model experimental results obtained from gene expression and capture a snapshot of the expression as well as the correlation of the experimental samples. Due to the fact that the noise to signal ratio in most biological databases are non-trivial, standard correlation networks may suffer from relatively high false-positive and false negative rates. Developing biologically-rich network enrichment algorithms can play a significant role in providing a healthy bias in the network and lead to the extraction of meaningful results. In addition, structure-based network filters can be used to reduce the network size and keep significant edges likely associated with strong biological signals. In this project, we propose the use of domain knowledge, not simply as an assessment tool, but as a basic component in building the correlation networks. We implemented a network integration algorithm that uses both gene expression data (experimental knowledge) and gene ontology data (domain knowledge) to build a biologically-rich correlation model. Our main hypothesis is that the integrated networks would reduce the harmful effects of outliers from imperfect data while maintaining the high concentration of network substructures that are likely to reveal novel, biologically-significant relationships. In addition, using the concept of “guilt by association”, we analyzed the clusters of the integrated networks and found that there was a significant increase of enrichment scores relative to the original networks. We also show higher concentration of known biological motifs calculated in the enriched networks. Based on the results obtained so far, the effects of outliers have been diminished in the new networks without the loss of the novel relationships.
Additional Information (Optional)
Winner of Best Undergraduate Oral Presentation