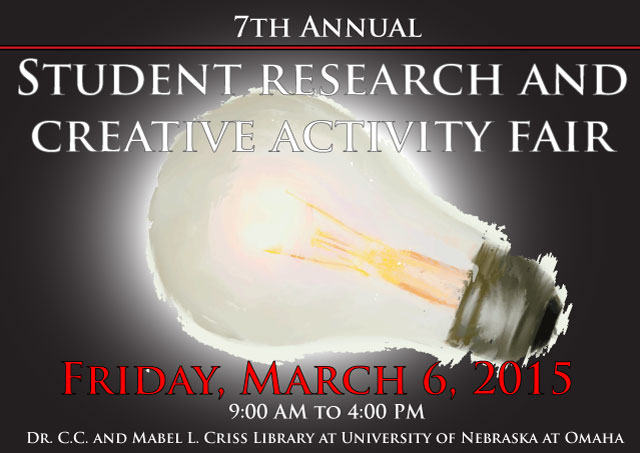
Distributed Decision Making with Rapidly Evolving Information using a Multi-agent System
Advisor Information
Prithviraj Dasgupta
Location
UNO Criss Library, Room 232
Presentation Type
Oral Presentation
Start Date
6-3-2015 3:00 PM
End Date
6-3-2015 3:15 PM
Abstract
We consider the problem of distributed decision making by a group of people, in the presence of rapidly evolving information, to determine the outcome of an undecided future event within a prescribed time window. Such decision making in the presence of rapidly arriving data are encountered in disasters, combat or battlefield scenarios, political or sports events, and even for group decisions around meeting project deadlines or fitness goals. Existing computational models of distributed decision making use multi- agent systems to model the interaction between people. Unfortunately, these opinion formation models are created under the assumption that no new information shows up over time. We hypothesize that a results-based update mechanism can effectively allow agents to adapt dynamically to new data as agents around them update their internal models based on this new data. To verify our hypothesis, we apply modern concepts in psychology with techniques in statistics, decision making under uncertainty, and multi-agent opinion formation to motivate a person to achieve a selected task.
Distributed Decision Making with Rapidly Evolving Information using a Multi-agent System
UNO Criss Library, Room 232
We consider the problem of distributed decision making by a group of people, in the presence of rapidly evolving information, to determine the outcome of an undecided future event within a prescribed time window. Such decision making in the presence of rapidly arriving data are encountered in disasters, combat or battlefield scenarios, political or sports events, and even for group decisions around meeting project deadlines or fitness goals. Existing computational models of distributed decision making use multi- agent systems to model the interaction between people. Unfortunately, these opinion formation models are created under the assumption that no new information shows up over time. We hypothesize that a results-based update mechanism can effectively allow agents to adapt dynamically to new data as agents around them update their internal models based on this new data. To verify our hypothesis, we apply modern concepts in psychology with techniques in statistics, decision making under uncertainty, and multi-agent opinion formation to motivate a person to achieve a selected task.