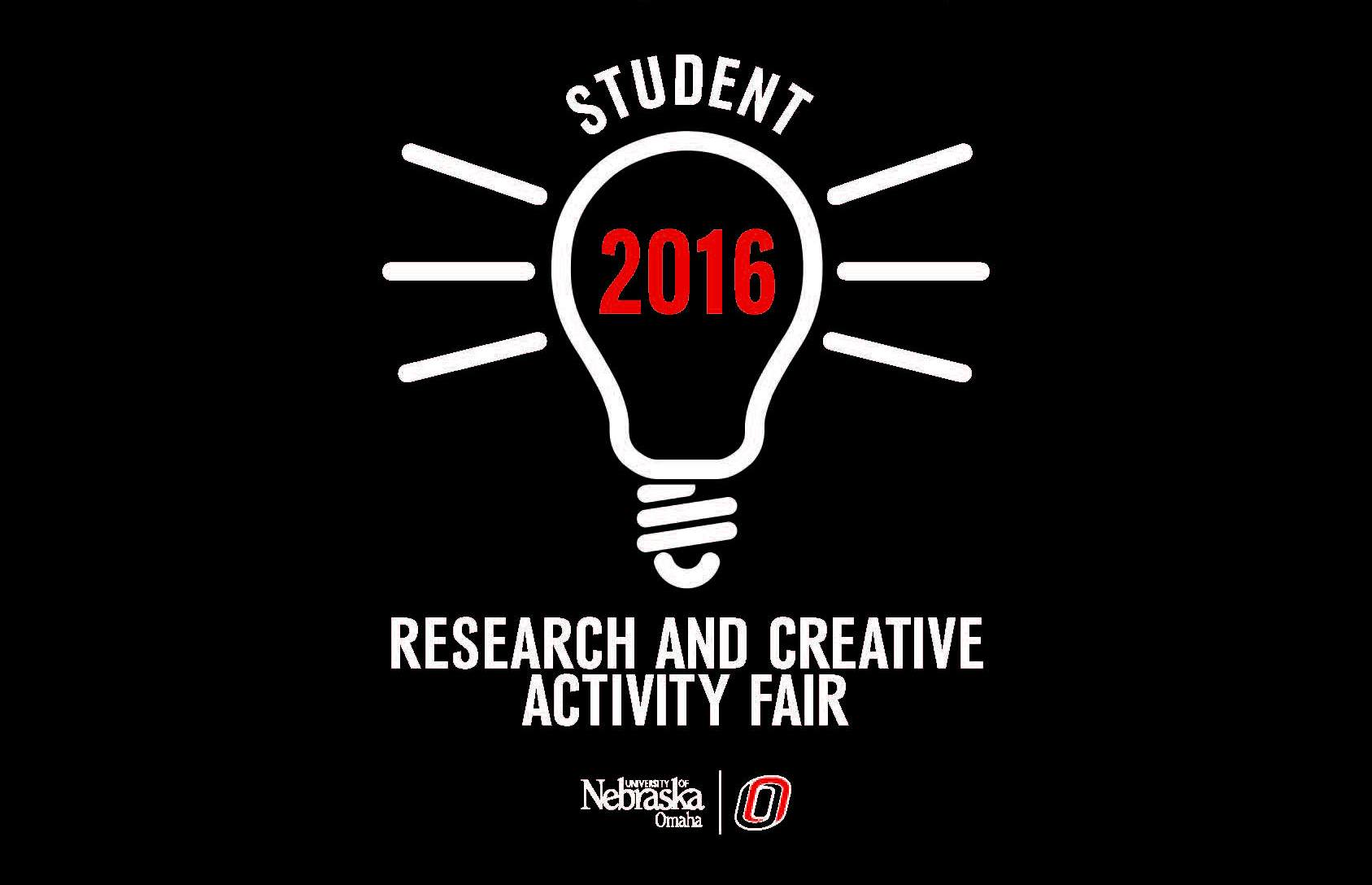
Data fusion techniques for information extraction from brain networks
Advisor Information
Hesham Ali
Location
UNO Criss Library, Room 232
Presentation Type
Oral Presentation
Start Date
4-3-2016 11:30 AM
End Date
4-3-2016 11:45 AM
Abstract
The advent of Brain Imaging technologies has allowed for non-invasive mapping of brain functionality.Functional Magnetic Resonance Imaging, Magnetoencephalography, and Diffusion Tensor Imaging each use biochemical movement to detect neuronal activity and anatomical structure. These advanced technologies drive the need for complex theories and thorough models. Perhaps the most impactful is the implementation of networks to model brain region interaction. Further, meta-research dictates the need for cross-experiment and cross-technology data fusion. Fusion between brain networks will extend these models for statistically powerful analysis, giving us the most formidable information possible about the human brain. However, the fusion of these models has unknown sensitivities. In this research, we explore granularity, an uncertainty term which has a strong influence on the information extraction of other biomedical data fusion models. We employ a graph theoretic model to analyze brain networks at various levels of granularity. In such models, hyper nodes are used to model subnetworks associated with potential brain functions. We define the qualities of the relationship between granularity and brain network fusion information extraction. Finally, we test our proposed model using datasets obtained the public database of BarinMap.
Data fusion techniques for information extraction from brain networks
UNO Criss Library, Room 232
The advent of Brain Imaging technologies has allowed for non-invasive mapping of brain functionality.Functional Magnetic Resonance Imaging, Magnetoencephalography, and Diffusion Tensor Imaging each use biochemical movement to detect neuronal activity and anatomical structure. These advanced technologies drive the need for complex theories and thorough models. Perhaps the most impactful is the implementation of networks to model brain region interaction. Further, meta-research dictates the need for cross-experiment and cross-technology data fusion. Fusion between brain networks will extend these models for statistically powerful analysis, giving us the most formidable information possible about the human brain. However, the fusion of these models has unknown sensitivities. In this research, we explore granularity, an uncertainty term which has a strong influence on the information extraction of other biomedical data fusion models. We employ a graph theoretic model to analyze brain networks at various levels of granularity. In such models, hyper nodes are used to model subnetworks associated with potential brain functions. We define the qualities of the relationship between granularity and brain network fusion information extraction. Finally, we test our proposed model using datasets obtained the public database of BarinMap.