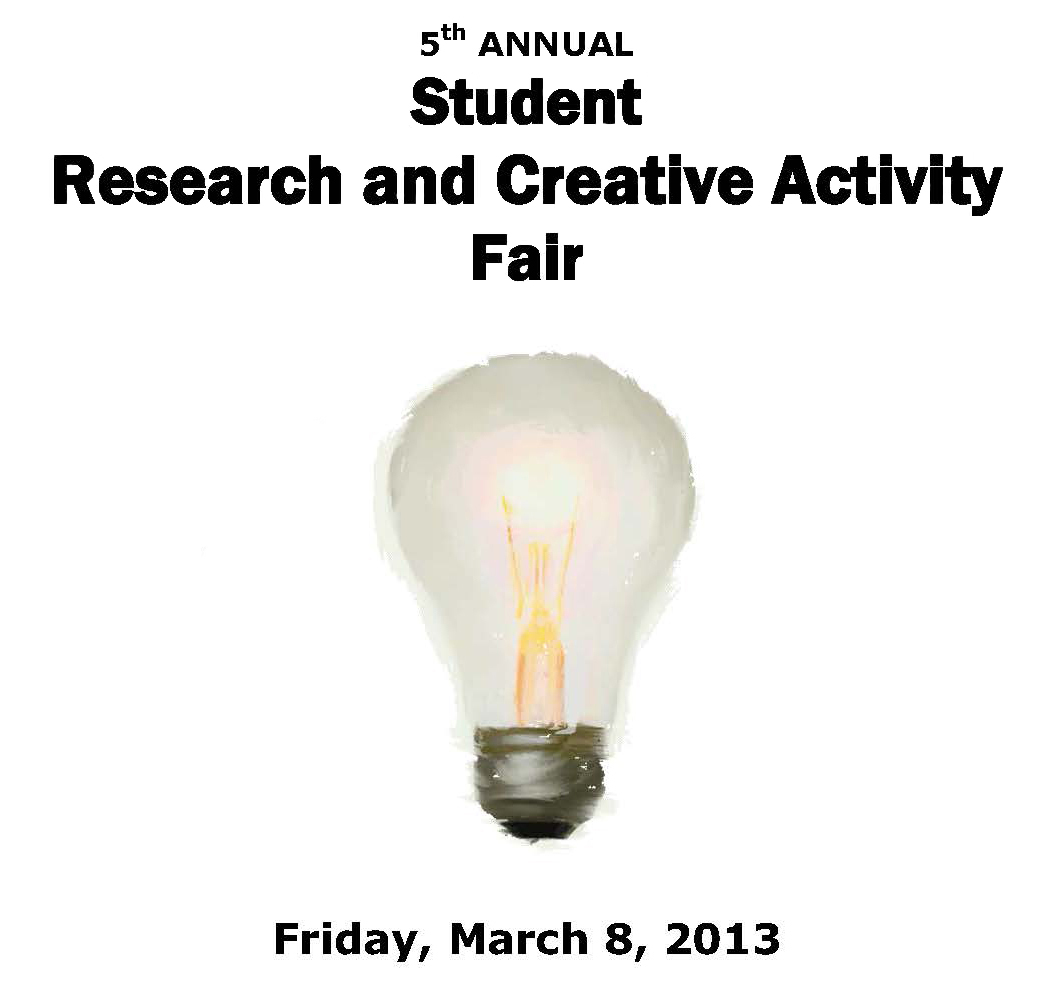
A Novel Approach for Integration of Association Rule Mining in the Analysis of GWAS for Cancer Networks
Advisor Information
Hesham Ali
Location
Milo Bail Student Center Council Room
Presentation Type
Oral Presentation
Start Date
8-3-2013 3:45 PM
End Date
8-3-2013 4:00 PM
Abstract
The advent of high throughput sequencing technologies have facilitated in generating a huge amount of genomic data. As a result, genome wide association studies (GWAS) have been able to identify a large number of strong associations between specific chromosomal loci and both Mendelian diseases and complex human disease. In addition, a wealth of genomic information in the form of publicly available databases is underutilized as a potential resource for uncovering functionally relevant markers underlying complex human traits. The discovery of genetic associations is an important factor in the understanding of human illness to derive disease pathways and a plethora of other information such as the disease-gene associations, the different types of variants associated with the diseases and their correlation with each other. Currently there is no model that can provide the correlation between genetic variants, genes and phenotypes, which serves as a motivation for this research. We propose a graph theoretic approach that models the intricate relationships between the variants, genes and phenotypes, and highlights the common features among the various relationships which can be further explored to extract meaningful features describing the observed behavior of genetic variants for a phenotype. We introduce two types of networks; Phenotype-Variant Network and Phenotype-Gene Network, and use the two networks to explore the shared genetic architecture of complex diseases from the Genetic Association Database. Furthermore, we analyze the networks and explored important subnetworks for the disease class ‘Cancer’ and used association rule mining on the database to give accuracy scores for the association.
A Novel Approach for Integration of Association Rule Mining in the Analysis of GWAS for Cancer Networks
Milo Bail Student Center Council Room
The advent of high throughput sequencing technologies have facilitated in generating a huge amount of genomic data. As a result, genome wide association studies (GWAS) have been able to identify a large number of strong associations between specific chromosomal loci and both Mendelian diseases and complex human disease. In addition, a wealth of genomic information in the form of publicly available databases is underutilized as a potential resource for uncovering functionally relevant markers underlying complex human traits. The discovery of genetic associations is an important factor in the understanding of human illness to derive disease pathways and a plethora of other information such as the disease-gene associations, the different types of variants associated with the diseases and their correlation with each other. Currently there is no model that can provide the correlation between genetic variants, genes and phenotypes, which serves as a motivation for this research. We propose a graph theoretic approach that models the intricate relationships between the variants, genes and phenotypes, and highlights the common features among the various relationships which can be further explored to extract meaningful features describing the observed behavior of genetic variants for a phenotype. We introduce two types of networks; Phenotype-Variant Network and Phenotype-Gene Network, and use the two networks to explore the shared genetic architecture of complex diseases from the Genetic Association Database. Furthermore, we analyze the networks and explored important subnetworks for the disease class ‘Cancer’ and used association rule mining on the database to give accuracy scores for the association.