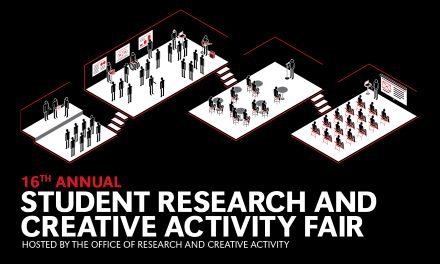
Graph Database for Mining of Multi-omics Data
Advisor Information
Hesham H. Ali
Location
Room 225
Presentation Type
Oral Presentation
Start Date
1-3-2019 9:00 AM
End Date
1-3-2019 10:15 AM
Abstract
Due to the advancement in high throughput technologies and robust experimental designs, more studies are incorporating multiple technologies to understand the molecular dynamics. These experiments produce wide variety of large amount of data spanning from genomics, transcriptomics, proteomics, and epigenetics. The multi-omics data are heterogeneous and come from different biological levels. Integration of multi-omics data to extract relevant biological information is currently a big challenge. This paper proposes a graph database model to efficiently store and mine multi-omics data. We show a working model of this graph database with transcriptomics, genomics, epigenetics and clinical data for three cancer types from the Cancer Genome Atlas. Moreover, we highlight the usefulness of graph database mining to extract relevant biological interpretations and also to find novel relationships between different data levels.
Graph Database for Mining of Multi-omics Data
Room 225
Due to the advancement in high throughput technologies and robust experimental designs, more studies are incorporating multiple technologies to understand the molecular dynamics. These experiments produce wide variety of large amount of data spanning from genomics, transcriptomics, proteomics, and epigenetics. The multi-omics data are heterogeneous and come from different biological levels. Integration of multi-omics data to extract relevant biological information is currently a big challenge. This paper proposes a graph database model to efficiently store and mine multi-omics data. We show a working model of this graph database with transcriptomics, genomics, epigenetics and clinical data for three cancer types from the Cancer Genome Atlas. Moreover, we highlight the usefulness of graph database mining to extract relevant biological interpretations and also to find novel relationships between different data levels.