Author ORCID Identifier
Files
Download Full Text (34.6 MB)
Description
This is an open access book.
Statistical machine learning (ML) has triggered a renaissance of artificial intelligence (AI). While the most successful ML models, including Deep Neural Networks (DNN), have developed better predictivity, they have become increasingly complex, at the expense of human interpretability (correlation vs. causality). The field of explainable AI (xAI) has emerged with the goal of creating tools and models that are both predictive and interpretable and understandable for humans.
Explainable AI is receiving huge interest in the machine learning and AI research communities, across academia, industry, and government, and there is now an excellent opportunity to push towards successful explainable AI applications. This volume will help the research community to accelerate this process, to promote a more systematic use of explainable AI to improve models in diverse applications, and ultimately to better understand how current explainable AI methods need to be improved and what kind of theory of explainable AI is needed.
After overviews of current methods and challenges, the editors include chapters that describe new developments in explainable AI. The contributions are from leading researchers in the field, drawn from both academia and industry, and many of the chapters take a clear interdisciplinary approach to problem-solving. The concepts discussed include explainability, causability, and AI interfaces with humans, and the applications include image processing, natural language, law, fairness, and climate science.
ISBN
978-3-031-04083-2
Publication Date
4-16-2022
Publisher
Springer
Keywords
Computer Science, informatics, conference proceedings, research, applications, open access
Recommended Citation
Holzinger, Andreas Ed.; Goebel, Randy Ed.; Fong, Ruth Ed.; Moon, Taesup Ed.; Muller, Klaus-Robert Ed.; Samek, Wojciech Ed.; and Tsai, Chun-Hua, "xxAI - Beyond Explainable AI" (2022). Information Systems and Quantitative Analysis Faculty Books and Monographs. 2.
https://digitalcommons.unomaha.edu/isqafacbooks/2
Creative Commons License
This work is licensed under a Creative Commons Attribution 4.0 License.
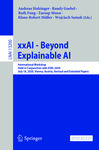
Files over 3MB may be slow to open. For best results, right-click and select "save as..."
Comments
University of Nebraska at Omaha's Dr. Chun-Hua Tsai co-authored the chapter titled Logic and pragmatics in AI Explanation, which can be directly accessed at https://digitalcommons.unomaha.edu/isqafacpub/134/
This book is open access, which means that you have free and unlimited access.
Explainable AI (xAI) aims to create tools and models that are predictive, interpretable, understandable for humans
Topic receiving huge interest in the machine learning and AI research communities
Contributions in this volume are from leading researchers in the field, drawn from both academia and industry
International Workshop, Held in Conjunction with ICML 2020, July 18, 2020, Vienna, Austria, Revised and Extended Papers