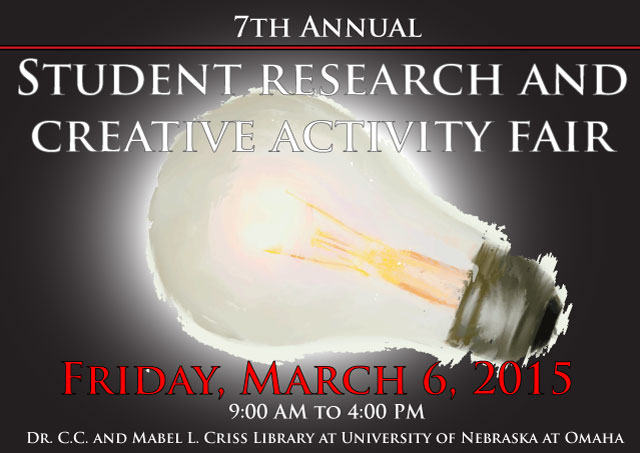
Big Data: How Correlation Networks can be used to Predict and Analyze Safety Problems
Advisor Information
Hesham Ali
Location
UNO Criss Library, Room 232
Presentation Type
Oral Presentation
Start Date
6-3-2015 2:30 PM
End Date
6-3-2015 2:45 PM
Abstract
Collecting extensive amounts of data from a variety of sources like sensors, scans or web services is no longer a technological issue. How to extract useful information is the step which most current research focuses on. This research takes another step forward and tries to find useful information out of large datasets, which the researcher might not even be aware of. Directly linked to the industry, this project intends to build up a correlation network out of data, related to the national bridges throughout Nebraska and potentially the entire USA. With the help of Graph theory and cluster analysis we want to find patterns to predict potential safety issues based on issues with similar bridges. This could help determining and prioritizing where to inspect the condition of bridges. This will save costs, increase the safety of the national railroad and road network and potentially save human lives. During regular inspections much data is collected which is used as an initial data source to find patterns helping to predict future problems and safety issues. Statics, traffic, bridge type, weather and bridge age are some examples of affecting variables. The data model is flexible enough to extend it at a later point with e.g. real-time sensor data. Ideally the final outcome will be an information systems which constantly reacts to data inputs in a supportive way. Visualizing the relevant information with the help of graphs, tables, filters and maps mark some of the features.
Big Data: How Correlation Networks can be used to Predict and Analyze Safety Problems
UNO Criss Library, Room 232
Collecting extensive amounts of data from a variety of sources like sensors, scans or web services is no longer a technological issue. How to extract useful information is the step which most current research focuses on. This research takes another step forward and tries to find useful information out of large datasets, which the researcher might not even be aware of. Directly linked to the industry, this project intends to build up a correlation network out of data, related to the national bridges throughout Nebraska and potentially the entire USA. With the help of Graph theory and cluster analysis we want to find patterns to predict potential safety issues based on issues with similar bridges. This could help determining and prioritizing where to inspect the condition of bridges. This will save costs, increase the safety of the national railroad and road network and potentially save human lives. During regular inspections much data is collected which is used as an initial data source to find patterns helping to predict future problems and safety issues. Statics, traffic, bridge type, weather and bridge age are some examples of affecting variables. The data model is flexible enough to extend it at a later point with e.g. real-time sensor data. Ideally the final outcome will be an information systems which constantly reacts to data inputs in a supportive way. Visualizing the relevant information with the help of graphs, tables, filters and maps mark some of the features.