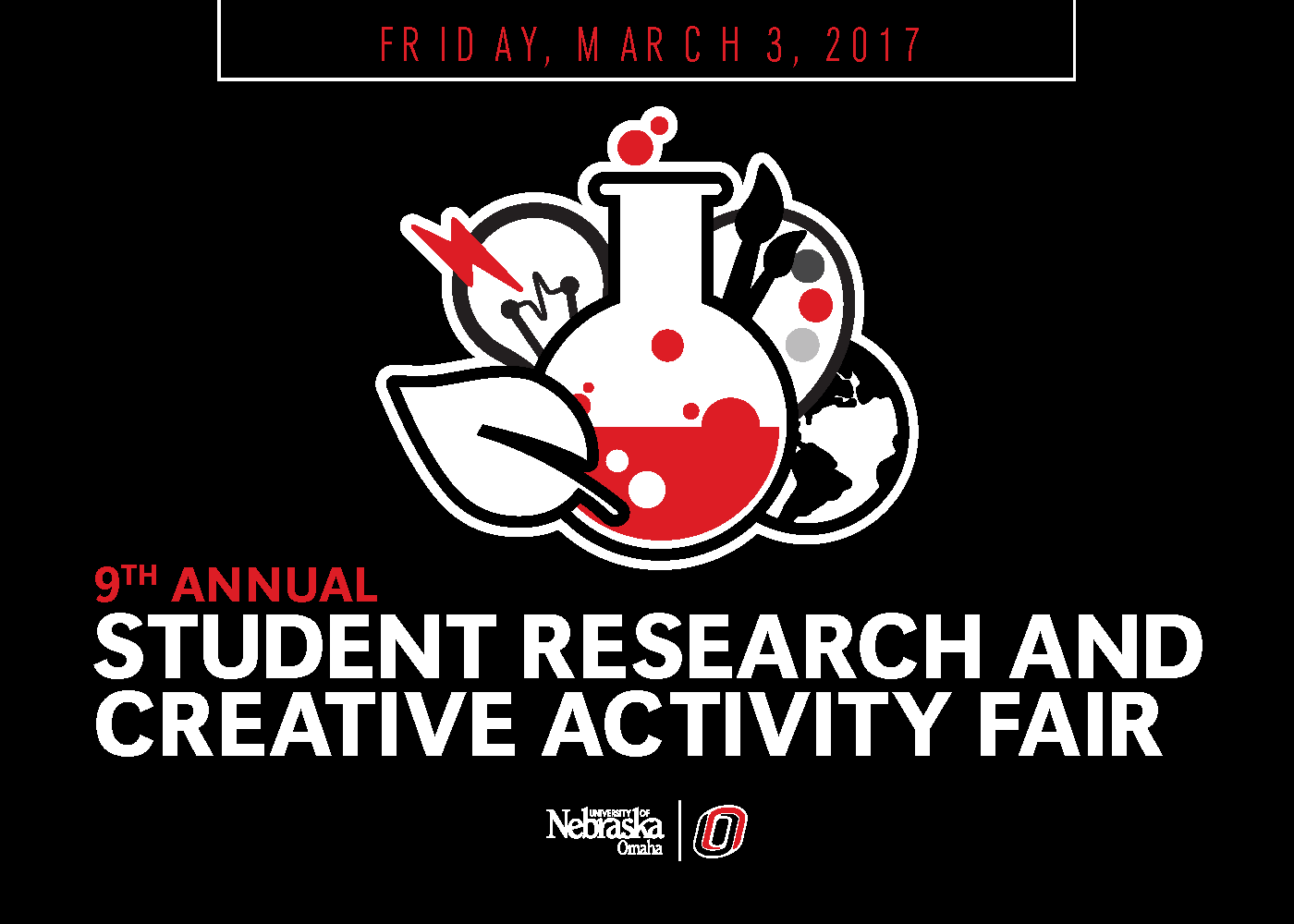
Real-time Robot Path Planning Around Complex Obstacle Patterns through Learning and Transferring Options
Advisor Information
Prithviraj Dasgupta
Location
UNO Criss Library, Room 225
Presentation Type
Oral Presentation
Start Date
3-3-2017 1:30 PM
End Date
3-3-2017 1:45 PM
Abstract
Extra-terrestrial navigation and path planning has been an area of substantial interest in the robotics community. For completely adopting an autonomous model, improved path planning techniques need to be developed which will invoke reliable navigation in an unfamiliar environment without human intervention and thus avoid communication delays. We consider the problem of robot path planning in an environment where the obstacle details are initially unknown but the robot can reuse relevant knowledge about collision avoidance learned from previous experience. We propose an algorithm that enables robots to improve their path planning capability by dynamically learning new environmental patterns and corresponding maneuvers from their past navigational experience and probabilistically predict the path to be adopted in presence of partial environmental information. We have conducted several simulated experiments and found that our algorithm takes 24% planning time and 39% total time to solve the same navigation problem compared to a recent, sampling-based path planner.
Real-time Robot Path Planning Around Complex Obstacle Patterns through Learning and Transferring Options
UNO Criss Library, Room 225
Extra-terrestrial navigation and path planning has been an area of substantial interest in the robotics community. For completely adopting an autonomous model, improved path planning techniques need to be developed which will invoke reliable navigation in an unfamiliar environment without human intervention and thus avoid communication delays. We consider the problem of robot path planning in an environment where the obstacle details are initially unknown but the robot can reuse relevant knowledge about collision avoidance learned from previous experience. We propose an algorithm that enables robots to improve their path planning capability by dynamically learning new environmental patterns and corresponding maneuvers from their past navigational experience and probabilistically predict the path to be adopted in presence of partial environmental information. We have conducted several simulated experiments and found that our algorithm takes 24% planning time and 39% total time to solve the same navigation problem compared to a recent, sampling-based path planner.