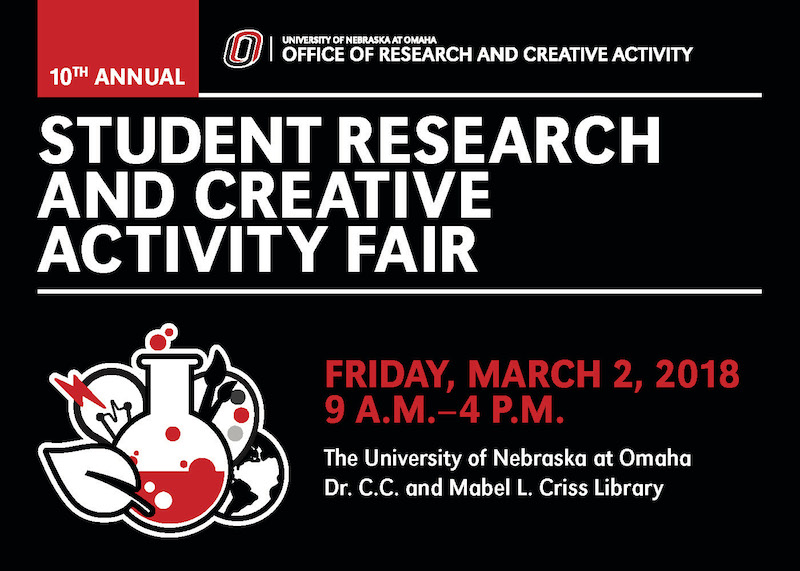
A rank-based temporal network approach to mine genes exhibiting significant expression variations
Advisor Information
Kate Cooper
Location
Dr. C.C. and Mabel L. Criss Library
Presentation Type
Poster
Start Date
2-3-2018 10:45 AM
End Date
2-3-2018 12:00 PM
Abstract
A networks is a structure that represents elements and their relationships. The study of biological networks can be used to understand a system of cellular elements. Ranking can be applied to a network in order to discover meaningful information from the network. Ranking has never been used to gather insights from in biological networks. In this paper, we propose a methodology for creating rank-based temporal network that can be used identify genes which are responsible for temporal shifts in cell expression patterns. Our network is made from gene expression data. The network is composed of genes as nodes and the correlation of their expression as edges. Through this methodology, we demonstrate that network modeling can be used to identify patterns and anomalies in gene expression data. We propose a rank-based system for identifying time-based anomalies and patterns, where for each network, nodes (genes) are ranked based on their degree (number of relationships), and then this rank is analyzed for change over time. Our research seeks to prove that the rank of high-degree nodes do not change rank over time and to find nodes that vary in degree ranking based on pleiotropy. By applying anomaly detection algorithms to the ranks of each node per network over time, we can identify which genes which are responsible for temporal shifts in in cell expression patterns. We expect the results to show that genes maintain the structure but not their connections.
A rank-based temporal network approach to mine genes exhibiting significant expression variations
Dr. C.C. and Mabel L. Criss Library
A networks is a structure that represents elements and their relationships. The study of biological networks can be used to understand a system of cellular elements. Ranking can be applied to a network in order to discover meaningful information from the network. Ranking has never been used to gather insights from in biological networks. In this paper, we propose a methodology for creating rank-based temporal network that can be used identify genes which are responsible for temporal shifts in cell expression patterns. Our network is made from gene expression data. The network is composed of genes as nodes and the correlation of their expression as edges. Through this methodology, we demonstrate that network modeling can be used to identify patterns and anomalies in gene expression data. We propose a rank-based system for identifying time-based anomalies and patterns, where for each network, nodes (genes) are ranked based on their degree (number of relationships), and then this rank is analyzed for change over time. Our research seeks to prove that the rank of high-degree nodes do not change rank over time and to find nodes that vary in degree ranking based on pleiotropy. By applying anomaly detection algorithms to the ranks of each node per network over time, we can identify which genes which are responsible for temporal shifts in in cell expression patterns. We expect the results to show that genes maintain the structure but not their connections.