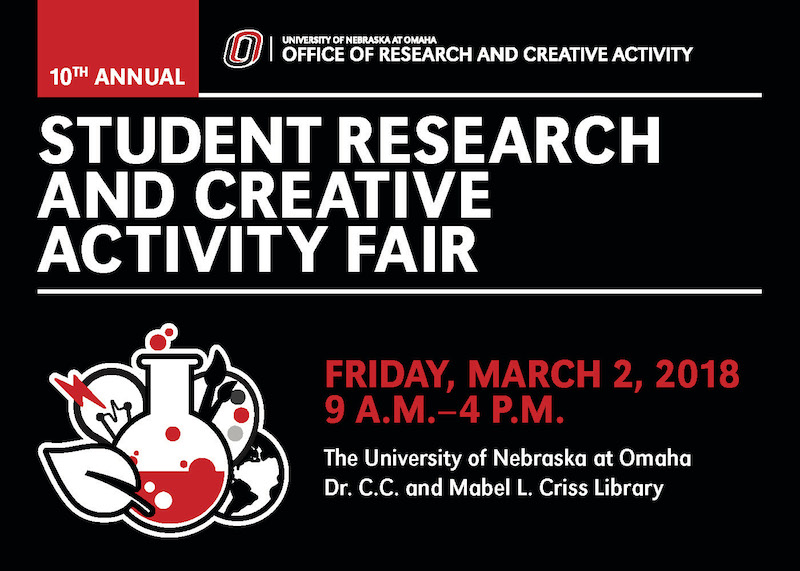
Real-time Robot Path Planning in Complex Environments Leveraging Learning from Past Experiences
Advisor Information
Dr. Raj Dasgupta
Location
UNO Criss Library, Room 232
Presentation Type
Oral Presentation
Start Date
2-3-2018 1:15 PM
End Date
2-3-2018 1:30 PM
Abstract
In this work, we consider the problem of robot path planning in an initially unknown environment where the robot does not have access to an a priori map of its environment but is aware of some common obstacle patterns along with the paths that enable it to circumnavigate around these obstacles. In order to autonomously improve its navigation performance, the robot should be able to identify significant obstacle patterns and learn corresponding obstacle avoidance maneuvers as it navigates through different environments in order to solve its tasks. To achieve this objective, we propose a novel online algorithm called Incremental State Discovery Via Clustering (ISDC) which enables a robot to dynamically determine important obstacle patterns in its environments and their best representations as combinations of initially available basic obstacle patterns. In order to assess the advantage of our approach, we have compared its performance with a previously proposed hierarchical navigation technique in the Webots commercial simulator using Coroware Corobot robot in different environments having various obstacle geometries and distribution where the robot had to perform a myriad of navigation tasks. Our results validate that clustering obstacle features using ISDC reduces the overall planning time by 81%, total time by 64%, total distance by 8% in comparison to a previously proposed hierarchical navigation technique.
Real-time Robot Path Planning in Complex Environments Leveraging Learning from Past Experiences
UNO Criss Library, Room 232
In this work, we consider the problem of robot path planning in an initially unknown environment where the robot does not have access to an a priori map of its environment but is aware of some common obstacle patterns along with the paths that enable it to circumnavigate around these obstacles. In order to autonomously improve its navigation performance, the robot should be able to identify significant obstacle patterns and learn corresponding obstacle avoidance maneuvers as it navigates through different environments in order to solve its tasks. To achieve this objective, we propose a novel online algorithm called Incremental State Discovery Via Clustering (ISDC) which enables a robot to dynamically determine important obstacle patterns in its environments and their best representations as combinations of initially available basic obstacle patterns. In order to assess the advantage of our approach, we have compared its performance with a previously proposed hierarchical navigation technique in the Webots commercial simulator using Coroware Corobot robot in different environments having various obstacle geometries and distribution where the robot had to perform a myriad of navigation tasks. Our results validate that clustering obstacle features using ISDC reduces the overall planning time by 81%, total time by 64%, total distance by 8% in comparison to a previously proposed hierarchical navigation technique.