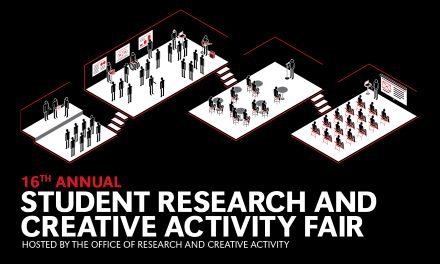
Advisor Information
Hesham Ali
Location
Criss Library 232
Presentation Type
Oral Presentation
Start Date
1-3-2019 9:00 AM
End Date
1-3-2019 10:15 AM
Abstract
Functional ability has been always considered as one of the important determining factors of individuals’ health and quality of life. Traditional movement analysis systems require expensive facilities and frequent visits for patients to specialized laboratories. Portability and affordability of wearable sensors along with their improved accuracy and capability of monitoring movement during daily activities make them a potential alternative for analyzing mobility patterns for clinical and health assessment purposes. Wearable-based movement data, when combined with other relevant clinical or laboratory data, could enhance evidence-based healthcare and data-driven Clinical Decision Support Systems (CDSS). Utilizing the data from wearable devices, many researchers have developed diagnostic/predictive models, including predictive models for fall detection, or diagnostic models for dyskinesia, bradykinesia, tremor, UPDRS score, and freezing of gait in patients with Parkinson’s disease. However, despite the wide usage of wearable-based gait analysis in the field of human movement research and its evident benefits to the healthcare domain, it is not still utilized in real-life settings nor leveraged via clinical decision support systems (CDSS). This is partially due to data quality issues, lack of accurate and robust models, lack of model interpretability, and lack of sophisticated visualization tools for continuous monitoring of individuals and their associated health parameters over time.
In this study, we propose a new approach to address these issues. The proposed solution uses a new Similarity Network model, a Document-of-Words coding scheme, a sophisticated feature selection algorithm and machine learning techniques to extract useful knowledge from data collected from wearable devises. Early results indicate that the proposed solutions provide an accurate assessment of health levels and can differentiate between healthy individuates and those with neurodegenerative conditions.
Files over 3MB may be slow to open. For best results, right-click and select "save as..."
Included in
Other Biomedical Engineering and Bioengineering Commons, Other Computer Engineering Commons
Mobility-Based Models for Advancing Diagnostic/Predictive Healthcare
Criss Library 232
Functional ability has been always considered as one of the important determining factors of individuals’ health and quality of life. Traditional movement analysis systems require expensive facilities and frequent visits for patients to specialized laboratories. Portability and affordability of wearable sensors along with their improved accuracy and capability of monitoring movement during daily activities make them a potential alternative for analyzing mobility patterns for clinical and health assessment purposes. Wearable-based movement data, when combined with other relevant clinical or laboratory data, could enhance evidence-based healthcare and data-driven Clinical Decision Support Systems (CDSS). Utilizing the data from wearable devices, many researchers have developed diagnostic/predictive models, including predictive models for fall detection, or diagnostic models for dyskinesia, bradykinesia, tremor, UPDRS score, and freezing of gait in patients with Parkinson’s disease. However, despite the wide usage of wearable-based gait analysis in the field of human movement research and its evident benefits to the healthcare domain, it is not still utilized in real-life settings nor leveraged via clinical decision support systems (CDSS). This is partially due to data quality issues, lack of accurate and robust models, lack of model interpretability, and lack of sophisticated visualization tools for continuous monitoring of individuals and their associated health parameters over time.
In this study, we propose a new approach to address these issues. The proposed solution uses a new Similarity Network model, a Document-of-Words coding scheme, a sophisticated feature selection algorithm and machine learning techniques to extract useful knowledge from data collected from wearable devises. Early results indicate that the proposed solutions provide an accurate assessment of health levels and can differentiate between healthy individuates and those with neurodegenerative conditions.