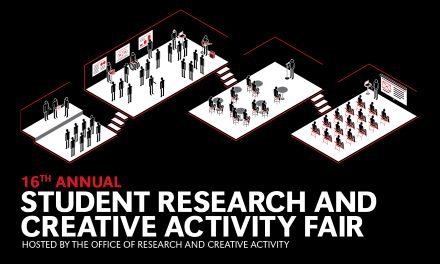
Assigning Risk Ranks to Civil Infrastructures using Big Data Analytics and Correlation Network Models
Advisor Information
Hesham H Ali
Location
MBSC 224
Presentation Type
Oral Presentation
Start Date
6-3-2020 9:00 AM
End Date
6-3-2020 10:15 AM
Abstract
It has been reported that over half the bridges around the world are not safe and nearly 20% of rural bridges in the United States are in serious conditions. Clearly, this is a major challenge that affects the economic growth and public safety. Various budget constraints and the scarcity in human resources to inspect bridges have always been trending problems, which motivate the research community to identify novel approaches to bridge health monitoring with the particular focus of identifying bridges that need immediate attention. National Bridge Inventory (NBI) database houses extensive data points that allows researchers to use some novel big data solutions to solve such problems on the available bridge data. In this study, we propose the use of correlation network model that are constructed based on the condition rating patterns. We show that analysis of such networks enables us to group bridges into various categories based on their deteriorating rates. Top ten clusters are identified by the Markov Clustering algorithm, and the risk ranks are assigned based on simple linear regression’s coefficients. Early results show that bridges in the highest risk rank cluster is deteriorating twice as fast as those in the slowest deteriorating group and that they may become structurally deficient in the near future. Hence, the authorities must prioritize their efforts and focus on the highest risk rank cluster as compared to following a routine inspection schedule.
Assigning Risk Ranks to Civil Infrastructures using Big Data Analytics and Correlation Network Models
MBSC 224
It has been reported that over half the bridges around the world are not safe and nearly 20% of rural bridges in the United States are in serious conditions. Clearly, this is a major challenge that affects the economic growth and public safety. Various budget constraints and the scarcity in human resources to inspect bridges have always been trending problems, which motivate the research community to identify novel approaches to bridge health monitoring with the particular focus of identifying bridges that need immediate attention. National Bridge Inventory (NBI) database houses extensive data points that allows researchers to use some novel big data solutions to solve such problems on the available bridge data. In this study, we propose the use of correlation network model that are constructed based on the condition rating patterns. We show that analysis of such networks enables us to group bridges into various categories based on their deteriorating rates. Top ten clusters are identified by the Markov Clustering algorithm, and the risk ranks are assigned based on simple linear regression’s coefficients. Early results show that bridges in the highest risk rank cluster is deteriorating twice as fast as those in the slowest deteriorating group and that they may become structurally deficient in the near future. Hence, the authorities must prioritize their efforts and focus on the highest risk rank cluster as compared to following a routine inspection schedule.