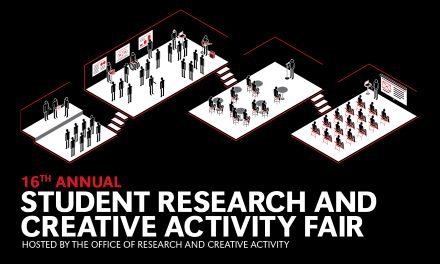
Accelerometer-based Gait Analysis for Elderly People using Deep Neural Networks
Advisor Information
Jong-Hoon Youn
Location
MBSC 224
Presentation Type
Oral Presentation
Start Date
6-3-2020 12:45 PM
End Date
6-3-2020 2:00 PM
Abstract
With the rapid growth of the elderly population, there has been considerable interest in preventing falls which are one of the major health risks of the elderly population. Many researchers have made great efforts to analyze elderly walking with the goal of preventing or predicting fall events. One of the studies determined that the rate of falls for the elderly was higher outdoors than indoors, and environmental factors such as slippery or unbalanced surfaces were greater risks. However, there is a lack of studies that analyze the gait characteristics of elderly people in various walking conditions. The objective of this study is to enhance our ability to understand elderly walking and fall risk by developing a method that utilizes wearable sensor gait characteristics to discriminate elderly’s walking between indoors and outdoors. In this study, we proposed a Deep Neural Network (DNN) approach for the classification of indoor and outdoor walk. The DNN was compared to traditional machine learning (ML) classifiers, such as support vector machine, k-nearest neighbor, decision tree, random forest, and logistic regression. Overall, the DNN classification outcomes were more accurate than the traditional ML classifiers. This could allow us to apply the emerging deep learning approach to accelerometer-based gait analysis.
Accelerometer-based Gait Analysis for Elderly People using Deep Neural Networks
MBSC 224
With the rapid growth of the elderly population, there has been considerable interest in preventing falls which are one of the major health risks of the elderly population. Many researchers have made great efforts to analyze elderly walking with the goal of preventing or predicting fall events. One of the studies determined that the rate of falls for the elderly was higher outdoors than indoors, and environmental factors such as slippery or unbalanced surfaces were greater risks. However, there is a lack of studies that analyze the gait characteristics of elderly people in various walking conditions. The objective of this study is to enhance our ability to understand elderly walking and fall risk by developing a method that utilizes wearable sensor gait characteristics to discriminate elderly’s walking between indoors and outdoors. In this study, we proposed a Deep Neural Network (DNN) approach for the classification of indoor and outdoor walk. The DNN was compared to traditional machine learning (ML) classifiers, such as support vector machine, k-nearest neighbor, decision tree, random forest, and logistic regression. Overall, the DNN classification outcomes were more accurate than the traditional ML classifiers. This could allow us to apply the emerging deep learning approach to accelerometer-based gait analysis.