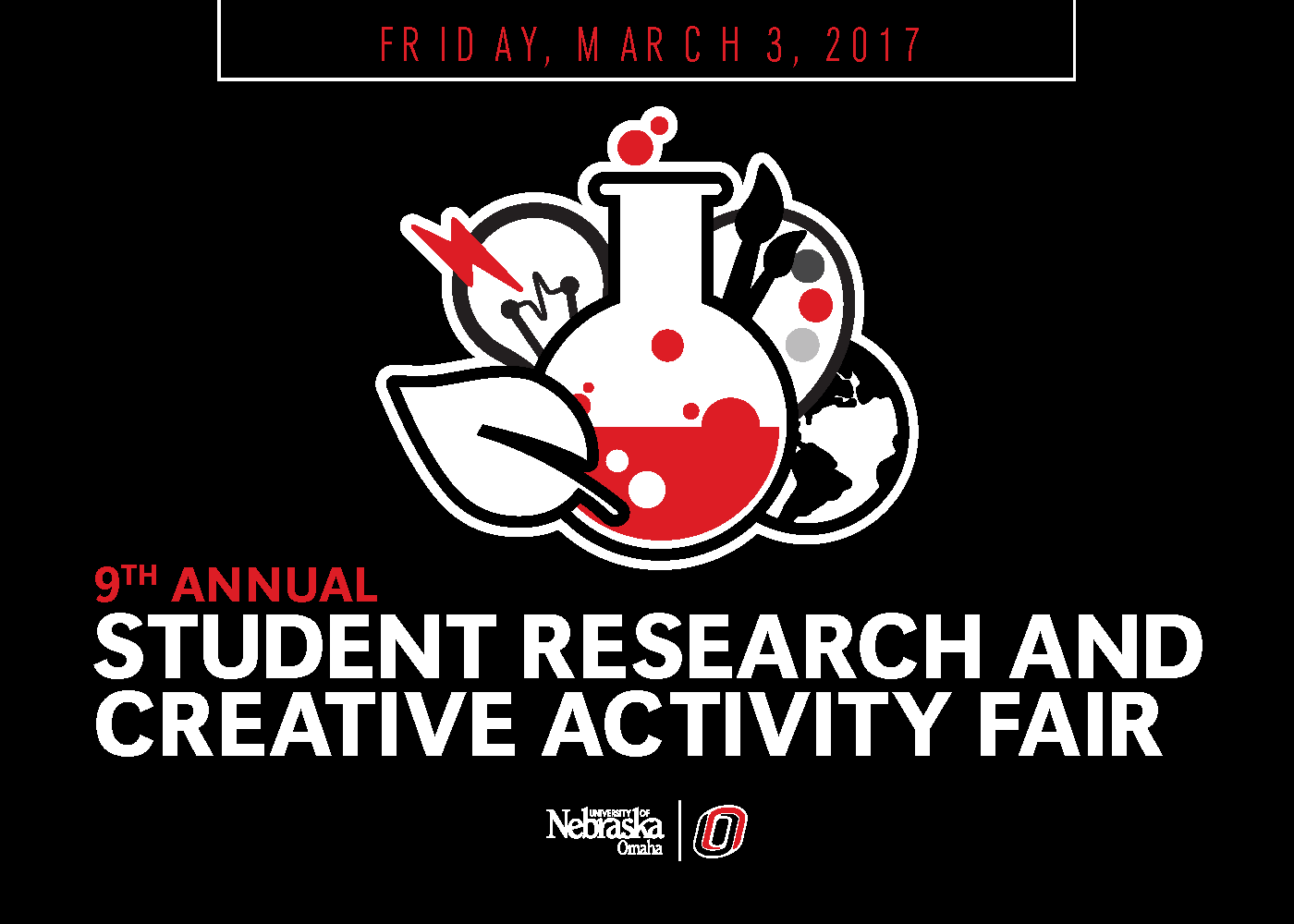
Advisor Information
Kathryn Cooper
Location
UNO Criss Library, Room 232
Presentation Type
Oral Presentation
Start Date
3-3-2017 10:30 AM
End Date
3-3-2017 10:45 AM
Abstract
Genes that share expression conditions show a biological correlation, and no modern method of visualization displays these intricate co-expression patterns better than a graph. Structural observations about a co-expression graph can reveal the secrets of the biological system that it models, but experimentally validated co-expression graphs are pain-staking work to produce. Present day correlation network analysis shows potential for drawing conclusions from large volumes of biological systems data in an inexpensive and easy-to-produce way, however, work remains to confirm the appropriateness and scope of such methods for specific, scientific application. Toward this effort, we generated a Pearson correlation network from gene expression data available to the public from the National Center for Biotechnology Information’s (NCBI) Gene Expression Omnibus (GEO) repository. From this data-set, we predicted shared transcription factor regulation among cliques of genes sharing upstream genomic motifs. Finally, our predictions, and thus the model itself, was contrasted against experimentally confirmed gene co-regulation data. Our process tested the hypothesis that correlation networks can guide the prediction of transcription factor co-regulation from gene expression and upstream sequence data with higher sensitivity and specificity than from this data alone, ultimately showing that our experimental results did not differentiate from a randomized control. Although this simplified method was unsuccessful, work remains to see if correlation networks can be constructed and normalized in a way that brings them closer to representing true co-expression.
Correlation Networks: Causative Relationships from Gene Expression Data
UNO Criss Library, Room 232
Genes that share expression conditions show a biological correlation, and no modern method of visualization displays these intricate co-expression patterns better than a graph. Structural observations about a co-expression graph can reveal the secrets of the biological system that it models, but experimentally validated co-expression graphs are pain-staking work to produce. Present day correlation network analysis shows potential for drawing conclusions from large volumes of biological systems data in an inexpensive and easy-to-produce way, however, work remains to confirm the appropriateness and scope of such methods for specific, scientific application. Toward this effort, we generated a Pearson correlation network from gene expression data available to the public from the National Center for Biotechnology Information’s (NCBI) Gene Expression Omnibus (GEO) repository. From this data-set, we predicted shared transcription factor regulation among cliques of genes sharing upstream genomic motifs. Finally, our predictions, and thus the model itself, was contrasted against experimentally confirmed gene co-regulation data. Our process tested the hypothesis that correlation networks can guide the prediction of transcription factor co-regulation from gene expression and upstream sequence data with higher sensitivity and specificity than from this data alone, ultimately showing that our experimental results did not differentiate from a randomized control. Although this simplified method was unsuccessful, work remains to see if correlation networks can be constructed and normalized in a way that brings them closer to representing true co-expression.