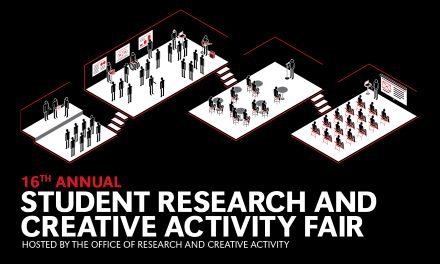
Advisor Information
Hesham Ali
Location
MBSC 224
Presentation Type
Oral Presentation
Start Date
6-3-2020 2:15 PM
End Date
6-3-2020 3:15 PM
Abstract
Investing in financial markets has continued to represent major challenges for many investors around the world. Attempting to understand the key aspects of stock markets, like when to buy or sell stocks, or which stocks are expected to perform well, remains extremely difficult. However, with the current availability of massive amounts of financial data associated with various markets over a long period of time, we finally have opportunities to apply the newly developed big data techniques and data-driven methodologies to analyze such complicated markets. As the available financial data continues to grow, clever approaches need to be employed to properly manage, structure and analyze such large data and provide some understanding to the behavior of the various entities of the financial markets. In this work, we propose new population analysis techniques that are based on the use of various network models to provide a manageable structure for this large data in ways that facilitate finding common patterns or similar behaviors. We conducted a pilot study on the stock market data for 3427 companies during the period 2000 - 2004. Given the financial parameters of these companies, we constructed various network models and employ population analysis methods for assessment and prediction purposes. As a starting point, we applied graph-based community algorithms to identify companies with similar features associated with their Access Return. While analyzing the top two communities, the obtained results showed that companies belong to the financial sector have the highest share in the market, and that companies with low levels of capitalization have high access returns. The preliminary analysis indicated that investing in companies with high capitalization did not guarantee high returns.
Innovative Population Analysis and Network Models for Analyzing Financial Markets
MBSC 224
Investing in financial markets has continued to represent major challenges for many investors around the world. Attempting to understand the key aspects of stock markets, like when to buy or sell stocks, or which stocks are expected to perform well, remains extremely difficult. However, with the current availability of massive amounts of financial data associated with various markets over a long period of time, we finally have opportunities to apply the newly developed big data techniques and data-driven methodologies to analyze such complicated markets. As the available financial data continues to grow, clever approaches need to be employed to properly manage, structure and analyze such large data and provide some understanding to the behavior of the various entities of the financial markets. In this work, we propose new population analysis techniques that are based on the use of various network models to provide a manageable structure for this large data in ways that facilitate finding common patterns or similar behaviors. We conducted a pilot study on the stock market data for 3427 companies during the period 2000 - 2004. Given the financial parameters of these companies, we constructed various network models and employ population analysis methods for assessment and prediction purposes. As a starting point, we applied graph-based community algorithms to identify companies with similar features associated with their Access Return. While analyzing the top two communities, the obtained results showed that companies belong to the financial sector have the highest share in the market, and that companies with low levels of capitalization have high access returns. The preliminary analysis indicated that investing in companies with high capitalization did not guarantee high returns.