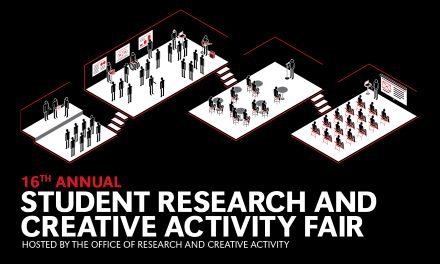
Evaluating Visual Search Performance in Glaucoma Patients Using Artificial Intelligence
Advisor Information
Dr. David Anderson and Dr. Deepak Khazanchi
Location
MBSC 224
Presentation Type
Oral Presentation
Start Date
6-3-2020 10:30 AM
End Date
6-3-2020 11:45 AM
Abstract
Glaucoma-related impairment in visual search performance is exacerbated when patients search within naturalistic relative to abstract scenes. Environmental scene context and image characteristics fundamentally influence patient performance during visual search tasks. To represent scene context we are using explainable artificial intelligence (XAI) techniques to define the complexity of an image in terms of the type and density of objects present within an image. Additionally, we are manually adding meta information (e.g. indoor vs outdoor environment) to the image in order to enhance our understanding of the scene and its effect on high-level experiential learning and memory while performing visual search. To explore the image characteristics, we are using computer vision algorithms to extract image properties like spatial frequency, contrast, brightness and sharpness. We will then compare the scene context and image characteristics between different groups, i.e. glaucoma patients, glaucoma suspects, and healthy controls to help us to identify which characteristics of a scene have the greatest impact on poor visual search.
Creative Commons License
This work is licensed under a Creative Commons Attribution 4.0 License.
Evaluating Visual Search Performance in Glaucoma Patients Using Artificial Intelligence
MBSC 224
Glaucoma-related impairment in visual search performance is exacerbated when patients search within naturalistic relative to abstract scenes. Environmental scene context and image characteristics fundamentally influence patient performance during visual search tasks. To represent scene context we are using explainable artificial intelligence (XAI) techniques to define the complexity of an image in terms of the type and density of objects present within an image. Additionally, we are manually adding meta information (e.g. indoor vs outdoor environment) to the image in order to enhance our understanding of the scene and its effect on high-level experiential learning and memory while performing visual search. To explore the image characteristics, we are using computer vision algorithms to extract image properties like spatial frequency, contrast, brightness and sharpness. We will then compare the scene context and image characteristics between different groups, i.e. glaucoma patients, glaucoma suspects, and healthy controls to help us to identify which characteristics of a scene have the greatest impact on poor visual search.