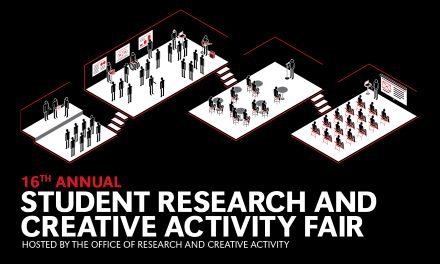
On Developing and Optimizing New Machine Learning Techniques to Analyze Mobility Parameters and Gait Patterns for Healthcare Purposes
Advisor Information
Hesham Ali
Location
MBSC 222
Presentation Type
Oral Presentation
Start Date
6-3-2020 9:00 AM
End Date
6-3-2020 10:15 AM
Abstract
With the explosive use of wearable devices, there is an urgent need to find impactful ways to utilize the data collected by such devices, particularly as it relates to health. It is not always clear which data points can be used to extract useful knowledge about the health of the individuals. In this work, network models and machine learning techniques are employed to analyze gait and mobility parameters extracted from data collected from the triaxle accelerometers attached to the ankles of individuals with Parkinson’s Disease, geriatrics, and healthy elderly. The main goal is to use data collected from wearble devices to accurately assess health levels and predict potential health hazards. With advanced technologies, it is tempting to collect more data. However, considering too much data for developing any predictive model may result in overfitting, which in turn leads to poor performance. Feature selection techniques allow researchers to remove data that is irrelevant or redundant, to avoid overfitting while reaching acceptable performance levels. In this study, we propose various future selection mechanisms to optimize the use of machine learning algorithms in processing mobility parameters used to assess health and aid healthcare providers in the diagnosis of conditions associated with mobility impairment. The result of the study indicates that variations of Generic Algorithms and Max_Information_Min_Correlation are the best performers among feature selection mechanisms regarding classification accuracy.
On Developing and Optimizing New Machine Learning Techniques to Analyze Mobility Parameters and Gait Patterns for Healthcare Purposes
MBSC 222
With the explosive use of wearable devices, there is an urgent need to find impactful ways to utilize the data collected by such devices, particularly as it relates to health. It is not always clear which data points can be used to extract useful knowledge about the health of the individuals. In this work, network models and machine learning techniques are employed to analyze gait and mobility parameters extracted from data collected from the triaxle accelerometers attached to the ankles of individuals with Parkinson’s Disease, geriatrics, and healthy elderly. The main goal is to use data collected from wearble devices to accurately assess health levels and predict potential health hazards. With advanced technologies, it is tempting to collect more data. However, considering too much data for developing any predictive model may result in overfitting, which in turn leads to poor performance. Feature selection techniques allow researchers to remove data that is irrelevant or redundant, to avoid overfitting while reaching acceptable performance levels. In this study, we propose various future selection mechanisms to optimize the use of machine learning algorithms in processing mobility parameters used to assess health and aid healthcare providers in the diagnosis of conditions associated with mobility impairment. The result of the study indicates that variations of Generic Algorithms and Max_Information_Min_Correlation are the best performers among feature selection mechanisms regarding classification accuracy.